The talk I was to give in Geneva (before weather sadly prevented my arrival) focused on “intertextuality beyond words” — I want to take this blog post to at least partially sketch out what I mean by that somewhat cryptic title. Most approaches to text reuse now, both in the IR world of identifying duplicate or near-duplicate documents, and in more humanistic approaches that explicitly focus on deliberate literary allusions (Lee 2007, Bamman and Crane 2008, Forstall et al. 2011, Büchler et al. 2012, Coffee et al. 2012) treat strings — i.e., sequences of characters, or words — as the atomic unit of comparison. Ovid’s Arma gravi numero violentaque bella parabam // edere is an allusion to Vergil’s Arma virumque cano for having several identical tokens, enclitics and rephrasings (parabam edere <-> cano) that are locally similar at the lexical and phrasal level. Lots of work in text reuse now focuses both on the fundamental problems of 1.) rigorously defining how we judge two things to be similar (an easy question for exact string matching but significantly harder for more distant but semantically similar pairs of text) and 2.) identifying examples of reuse at scale, when N2 brute-force comparisons is simply not feasible (Smith et al. 2013).
In thinking about “intertextuality beyond words,” I want to take a step back and think first about a much more fundamental question on which all text reuse depends: what is it we want to compare? Strings are only one example of a comparandum; in fact, we might think of them as occupying one far end of a continuum, where document-level comparisons (such as might be occasioned by a topic model) lie at the other end. String comparisons allow us to ask: is the Moon’s resplendent globe (Paradise Lost 4.723) an allusion to lucentumque globum Lunae (Aen. 6.725)? It enables exact comparison, and high precision is often possible. This is a very fine-grained comparison, in which we explicitly compare a sequence of words in one text with a sequence in another, possibly with some local transformations (e.g. using manual or inferred synonyms) if the search space is small enough. A topic model in contrast allows us to make coarser comparisons at the passage or document level: is the Aeneid topically about similar kinds of things as the Odyssey? (e.g., both may involve “war” and “journeys” in the same degree).
It’s the middle ground between these two that I think is the most interesting, and I think it may be a powerful area in which to think about text reuse. Between strings and topic models lies higher-level latent structure in text, coarser than that of an individual string but much finer than that of an entire document. Two examples of this kind of middle ground are representations of event structure and representations of character structure.
One example of event structure is that of semantic frames, which originated in a flurry of activity in the mid-1970s (Minksy 1974, Schank and Abelson 1975, Fillmore 1975, Rumelhardt 1975, Goffman 1975, Tannen 1979, Entman 1993) and have seen a resurgence in computational models today (Modi et al. 2012, O’Connor 2013, Cheung et al . 2013, Chambers 2013). A frame-semantic representation decomposes a predicate into its core arguments: “I bought a car from you”, “You sold a car to me” and “Sie verkauft mir ein Auto” all contain the same semantic arguments of Buyer, Goods, and Seller, though each are realized differently in each sentence; all denote the same abstract event (an instance of a commercial transaction).

Parallel frame semantic representation of the same commercial transaction in English and German.
Frame semantic methods are a very active research area in NLP right now, both in the supervised setting (Das 2012) and in the unsupervised one as well (i.e., not just assigning a frame-semantic parse to text, but learning what constitutes an appropriate set of frames and arguments at the same time). The application to text reuse is not far-fetched — in providing a level of abstraction over the individual words in a text, and seeing words as simply an instantiation of larger concepts that bear a predictable relationship to each other, they may be worth pursuing for finding parallels between texts that describe similar actions — e.g., where do two characters in different texts commit violent acts? Where do cities fall?
Representations of event structure naturally position an action — a predicate — as central to the description. An analogue of this are representations of text in which people are central to the representation — what we might think of as “character-centric NLP.” There are many ways we can group characters into coherent, and often overlapping, clusters — group membership like “policemen” and “firefighter” are common everyday abstractions by which we generalize about specific individuals by their vocational identities; “king” and “warrior” are similar “vocational” groups found in older texts. Thinking about how two characters are similar — perhaps defined by the actions they take, the actions they have done to them, and the means by which they are described (as in Bamman et al. 2013) — provides a level of abstraction above the unit of individual words and phrases, but below the document, in which we might think to mine examples of literary allusions. Byronic heros and gothic villains may be common throughout entire genres, but one focused example of “character reuse” are cases like H. Rider Haggard’s adventurer Allen Quartermain — here looking above the fine grain of words and into the totality of a character’s actions may reveal clear similarities to Indiana Jones.

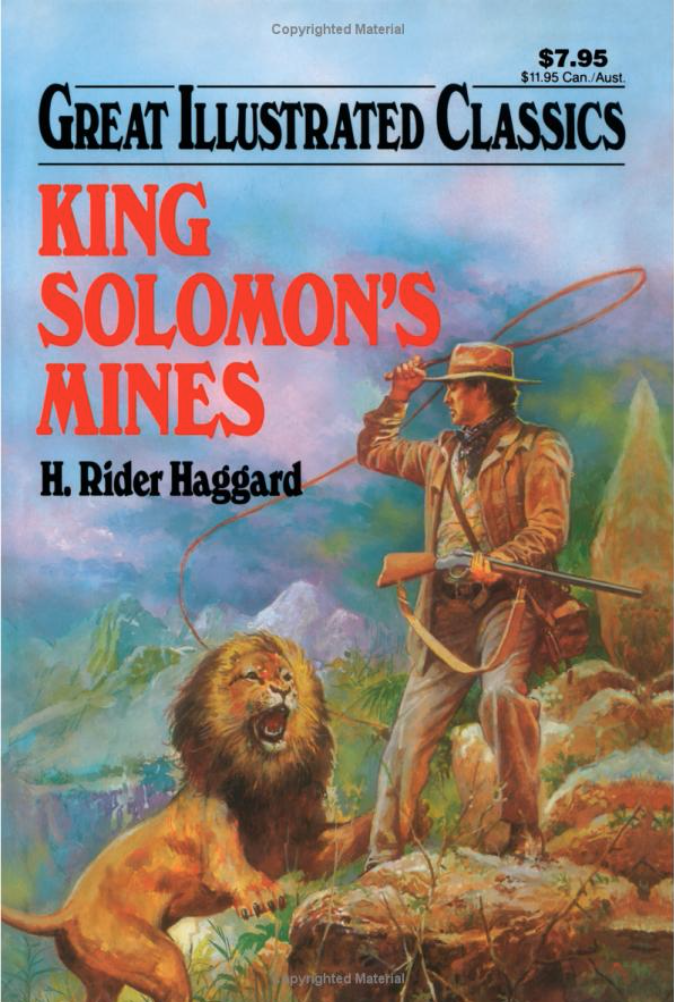
H. Rider Haggard’s King Solomon’s Mines.
Individual words and phrases are natural targets for text reuse; they are clearly one of the most salient means by which we hear echoes of one text in another. What I would argue is we needn’t presume that our methods for identifying reuse ends with them. Literary texts are not web pages or other classic targets of duplicate detection; in thinking about how they differ structurally from that genre of texts, we can bring entirely new methods to bear on this problem that exploit what makes them unique. Approaches to literary reuse that leverage paralinguistic information like meter (Forstall et al. 2011) are already one step in this direction; methods that look for similarities in higher-level event and character structure might be another promising step worth taking.
References
David Bamman, Brendan O’Connor, and Noah Smith. 2013. Learning latent personas of film characters. Proceedings of the Annual Meeting of the Association for Computational Linguistics.
David Bamman and Gregory Crane. 2008. The logic and discovery of textual allusion. Proceedings of the 2008 LREC Workshop on Language Technology for Cultural Heritage Data (LaTeCH 2008).
Marco Büchler, Gregory Crane, Maria Moritz and Alison Babeu. 2012. Increasing recall for text re-use in historical documents to support research in the humanities. Theory and Practice of Digital Libraries 2012.
Nathanael Chambers. 2013. Event schema induction with a probabilistic entity-driven model. Proceedings of the 2013 Conference on Empirical Methods in Natural Language Processing, pages 1797- 1807, Seattle, Washington, USA, October. Association for Computational Linguistics.
Jackie Chi Kit Cheung, Hoifung Poon, and Lucy Vanderwende. 2013. Probabilistic frame induction. Proceedings of the 2013 Conference of the North American Chapter of the Association for Computational Linguistics: Human Language Technologies, pages 837-846, Atlanta, Georgia, June. Association for Computational Linguistics.
N. Coffee, J.-P. Koenig, S. Poornima, C. Forstall, R. Ossewaarde, and S. Jacobson. 2012. Intertextuality in the digital age. Transactions of the American Philological Association 142.2: 383-422
Dipanjan Das. 2012. Semi-Supervised and Latent-Variable Models of Natural Language Semantics. Ph.D. thesis, Carnegie Mellon University.
Robert M. Entman. 1993. Framing: Toward clarification of a fractured paradigm. Journal of Communication, 43(4):51-58.
Charles Fillmore. 1975. An alternative to checklist theories of meaning. Proceedings of the First Annual Meeting of the Berkeley Linguistics Society. An alternative to checklist theories of meaning.
C. Forstall, S. Jacobson, and W. Schierer. 2011. Evidence of intertextuality: Investigating Paul the Deacon’s Angustae Vitae. Literary and Linguistic Computing 26 (3): 285-296.
Erving Goffman. 1974. Frame Analysis: An Essay on the Organization of Experience. Harper and Row, New York.
John Lee. 2007. A computational model of text reuse in ancient literary texts. ACL.
Marvin Minsky. 1974. A framework for representing knowledge. Technical report, MIT-AI Laboratory Memo 306.
Ashutosh Modi, Ivan Titov, and Alexandre Klementiev. 2012. Unsupervised induction of frame-semantic representations. Proceedings of the NAACL-HLT Workshop on the Induction of Linguistic Structure, WILS ’12, pages 1-7, Stroudsburg, PA, USA. Association for Computational Linguistics.
Brendan O’Connor. 2013. Learning frames from text with an unsupervised latent variable model. ArXiv, abs/1307.7382.
D.E. Rumelhart. 1975. Notes on a schema for stories. Representation and Understanding: Studies in Cognitive Science, pages 185-210.
Roger C. Schank and Robert P. Abelson. 1975. Scripts, plans, and knowledge. Proceedings of the 4th International Joint Conference on Artificial Intelligence Volume 1, IJCAI’75, pages 151-157, San Francisco, CA, USA. Morgan Kaufmann Publishers Inc.
David A. Smith, Ryan Cordell, and Elizabeth Maddock Dillon. 2013. Infectious texts: Modeling text reuse in nineteenth-century newspapers. IEEE Workshop on Big Data and the Humanities.
Deborah Tannen. 1979. What’s in a frame? surface evidence for underlying expectations. New Directions in Discourse Processing, pages 137-181, Norwood, NJ. Ablex.